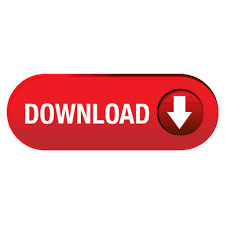
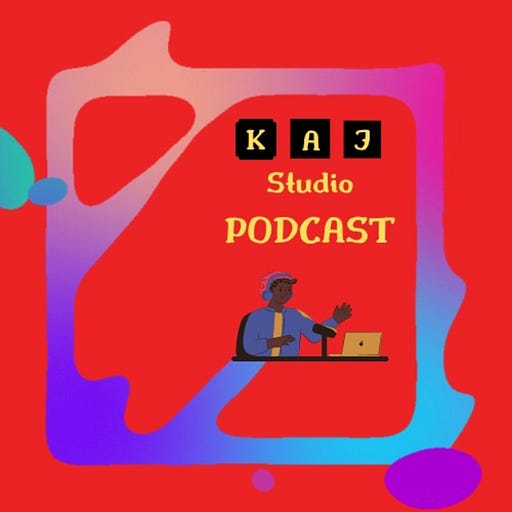
We further study the impact of different sensors and feature sets on performance and discuss the significant potential but also remaining challenges of forecasting user attention during mobile interactions. We demonstrate the feasibility of forecasting bidirectional attention shifts between the device and the environment as well as for predicting the first and total attention span on the device and environment using our method. As a first step towards a fully-fledged attention forecasting interface, we further propose a proof-of-concept method that uses device-integrated sensors and body-worn cameras to encode rich information on device usage and users' visual scene. To facilitate the development and evaluation of methods for attention forecasting, we present a novel long-term dataset of everyday mobile phone interactions, continuously recorded from 20 participants engaged in common activities on a university campus over 4.5 hours each (more than 90 hours in total). To address these limitations, we study attention forecasting - the challenging task of predicting whether users' overt visual attention (gaze) will shift between a mobile device and environment in the near future or how long users' attention will stay in a given location. after shifts have already happened, thereby severely limiting the adaptation capabilities and user experience. Users' visual attention is highly fragmented during mobile interactions but the erratic nature of these attention shifts currently limits attentive user interfaces to adapt after the fact, i.e. In addition we provide insights how this can be exploited in future work. We establish that there is a high correlation between expected task required skill level and the captured hand eye coordination of expert factory workers and that hand eye coordination can be used to distinguish between fine and gross motor skills. This setting consists of an up to 22 tasks assembly processes of two variants of a high quality product. To do so we investigate hand-eye coordination on precision tasks, its relation to fine and gross motor skills, in an unconstrained industrial setting. In this work, we investigate how we can utilize how humans behave in relation to task-required skills levels. While human workers can produce with a least with the same quality as machines do, they are not that consistent, so it's better to combine strengths of both men and machines. It does not store any personal data.Companies are re-focusing and making use of human labor in order to create individualized lot-size-1 products and not produce the exact same mass product again and again. The cookie is set by the GDPR Cookie Consent plugin and is used to store whether or not user has consented to the use of cookies. The cookie is used to store the user consent for the cookies in the category "Performance". This cookie is set by GDPR Cookie Consent plugin. The cookie is used to store the user consent for the cookies in the category "Other. The cookies is used to store the user consent for the cookies in the category "Necessary".
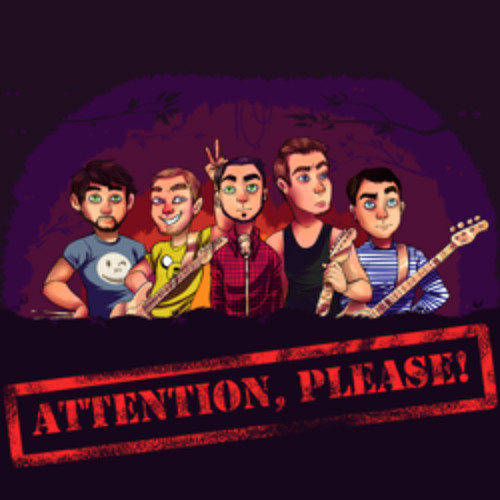
The cookie is set by GDPR cookie consent to record the user consent for the cookies in the category "Functional". The cookie is used to store the user consent for the cookies in the category "Analytics".
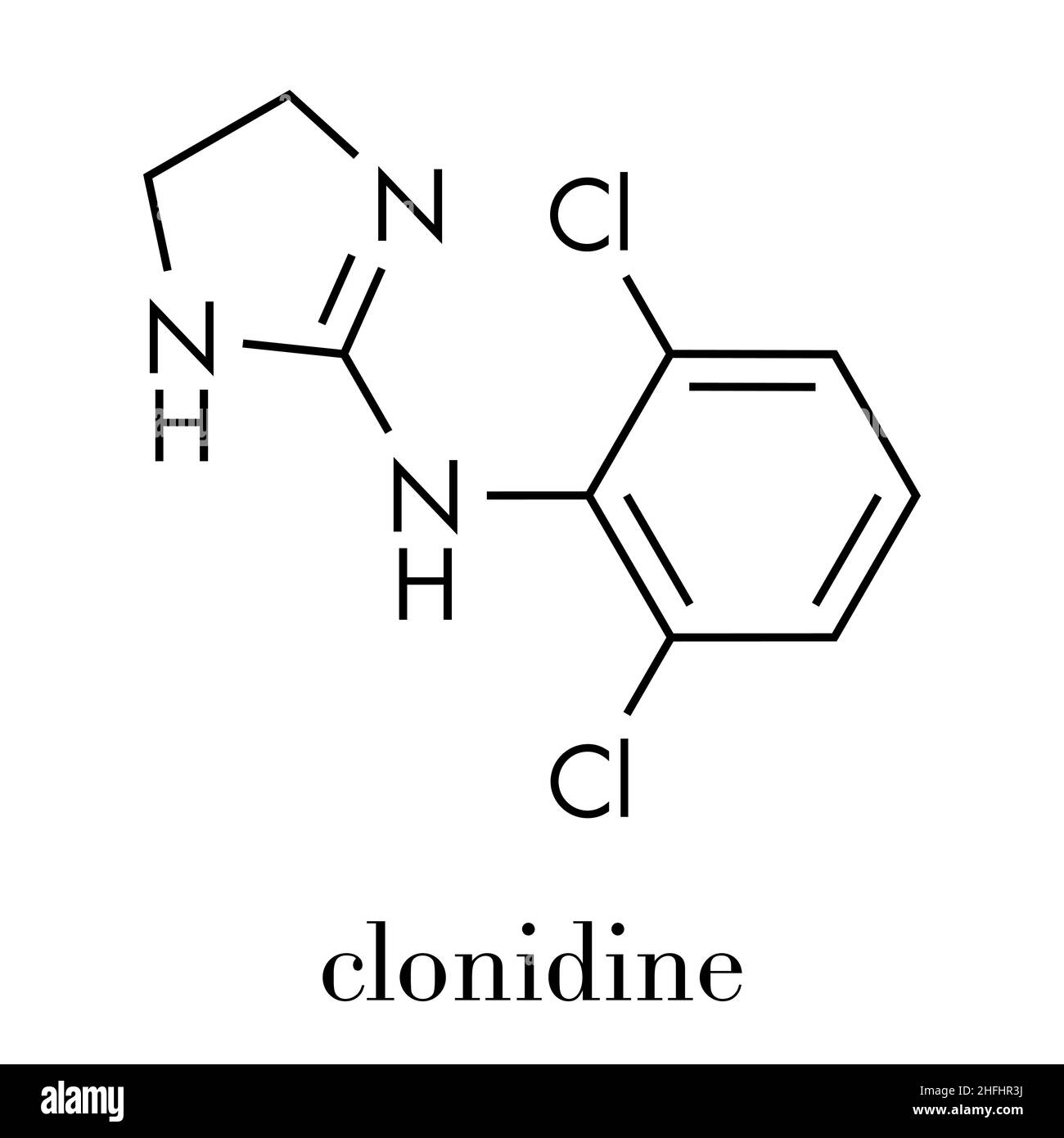
These cookies ensure basic functionalities and security features of the website, anonymously. Necessary cookies are absolutely essential for the website to function properly.
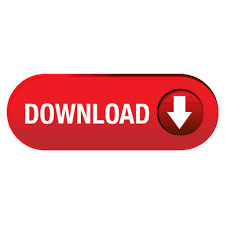